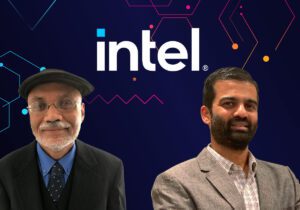
Close-to-the-metal architecture handles millions of OPS with predictable single-digit millisecond latencies.
Learn MoreClose-to-the-metal architecture handles millions of OPS with predictable single-digit millisecond latencies.
Learn MoreScyllaDB is purpose-built for data-intensive apps that require high throughput & predictable low latency.
Learn MoreLevel up your skills with our free NoSQL database courses.
Take a CourseOur blog keeps you up to date with recent news about the ScyllaDB NoSQL database and related technologies, success stories and developer how-tos.
Read MoreApache® and Apache Cassandra® are either registered trademarks or trademarks of the Apache Software Foundation in the United States and/or other countries. Amazon DynamoDB® and Dynamo Accelerator® are trademarks of Amazon.com, Inc. No endorsements by The Apache Software Foundation or Amazon.com, Inc. are implied by the use of these marks.